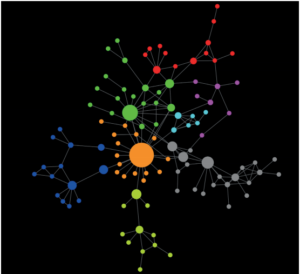
How the New Science of Computational History Is Changing the Study of the Past
How the New Science of Computational History Is Changing the Study of the Past
Applying network theory to medieval records suggests that historical events are governed by “laws of history”, just as nature is bound by the laws of physics.
One of the curious features of network science is that the same networks underlie entirely different phenomena. And as a result, these phenomena have deep similarities that are far from obvious at first glance. Good examples include the spread of disease, the size of forest fires and even the distribution of earthquake magnitude, which all follow a similar pattern. This is a direct result of them all sharing the same network structure.
So it’s usually no surprise that the same “laws” emerge when physicists find the same networks underlying other phenomenon. Exactly this has happened repeatedly in the social sciences. Network science has suddenly allowed social scientists to model societies, to study the way ideas, gossip, fashions and so on, flow through society and even to study how this influences opinion.
To do this they’ve used the tools developed to study other disciplines. That’s why the new field of computational social science has become so powerful so quickly.
But there’s another field of endeavour that also stands to benefit—the study of history. Throughout history, humans have formed networks that have played a profound role in the way events have unfolded. Historians have recently begun to reconstruct these networks using historical sources such as correspondence contemporary records and so on.
Today, Johannes Preiser-Kapeller at the Austrian Academy of Science in Vienna explains how this approach is casting a new light on various historical events. Indeed, the work has uncovered previously unknown patterns in the way history unfolds. In the same way that patterns in nature reveal the laws of physics, these discoveries are revealing the first laws of history.
Preiser-Kapeller has focused on medieval conflicts and particularly those relating to the Byzantine Empire in the 14th century, which was concentrated around Constantinople and its role as the link between European and Asian trade networks. This was a period of significant conflict due to changing political forces but also due to climate change caused by a small ice age during the middle ages and also by the spread of plague.
Preiser-Kapeller has reconstructed the political networks that existed at the time using surviving correspondence and other historical records. In these networks, each influential individual is a node with links drawn between those who share significant relationships. To be registered on the network, these links have to be recorded in correspondence with phrases such as My noble aunt or My imperial cousin. He also records how these change over time.
Preiser-Kapeller used standard algorithms to study various measures of network structure. These algorithms find clusters within the network, identify the most important actors in a network, and examine how individuals cluster around others who are similar in some way.
How these measures change over time turns out to have an important link to the major events that later unfold. For example, Preiser-Kapeller says the fragmentation of the political network created the conditions ripe for civil war which duly happened and permanently weakened the Byzantine Empire, which eventually collapsed in 1453.
These changes also followed some interesting patterns. “The distribution of frequencies of the number of conflict ties activated in a year tends to follow a power law,” says Preiser-Kapeller. Exactly the same power law patterns emerge when complexity scientists study the size distribution of wars, epidemics and religions.
An interesting question is whether the same patterns turn up elsewhere in history. To find out, compared the Byzantium network with those from five other periods of medieval conflict in Europe, Africa and Asia.
And the results make for interesting reading. “On average across all five polities, a change of ruler in one year increased the probability for another change in the following year threefold,” says Preiser-Kapeller. So the closer you are to an upheaval, the more likely there is to be another one soon. Or in other words, upheavals tend to cluster together.
That’s a rule that should sound familiar to geophysicists. A similar phenomenon exists in earthquake records—the more recent a big earthquake, the greater the likelihood of another big one soon. This is known as Omori’s law—that earthquakes tend to cluster together.
It’s no surprise that similar effects arise in these systems since they are both governed by the same network science. Historians would be well within their rights to adopt this and other patterns as “laws of history”.
These laws are ripe for further study. While there have been decades of study into the complexity that arises from network theory in many areas of science, there has been almost none in the field of history. That suggests there is significant low hanging fruit to be had by the first generation of computational historians, like Preiser-Kapeller. Expect to hear more about it the near future.
Ref: arxiv.org/abs/1606.03433 : Calculating the Middle Ages? The Project »Complexities and Networks in the Medieval Mediterranean and the Near East
Leave a Reply